Estudios originales
← vista completaPublicado el 17 de junio de 2024 | http://doi.org/10.5867/medwave.2024.05.2781
Eficiencia y comparabilidad del uso de nuevas plataformas de evidencia para la actualización de recomendaciones: experiencia con una guía de diabetes tipo 2 en Colombia
Efficiency and comparability of using new evidence platforms for updating recommendations: Experience with a type-2 diabetes guideline in Colombia
Resumen
Introducción La actualización de recomendaciones de las guías de práctica clínica requiere búsquedas bibliográficas exhaustivas y eficientes. Aunque están disponibles nuevas plataformas de información para grupos desarrolladores, su contribución a este propósito sigue siendo incierta.
Métodos Como parte de una revisión/actualización de 8 recomendaciones basadas en evidencia seleccionadas sobre diabetes tipo 2, evaluamos las siguientes cinco aproximaciones de búsqueda bibliográfica (dirigidas a revisiones sistemáticas, utilizando criterios predeterminados): PubMed para MEDLINE; Epistemonikos utilizando una búsqueda básica; Epistemonikos utilizando una estrategia de búsqueda estructurada; plataforma (L.OVE) y TRIP . Tres revisores clasificaron de forma independiente las referencias recuperadas como definitivamente o probablemente elegibles/no elegibles. Aquellas clasificadas en las mismas categorías "definitivas" para todos los revisores, se etiquetaron como "verdaderas" positivas/negativas. El resto se sometieron a una nueva evaluación y, si se consideraban por consenso elegibles/no elegibles, se convirtieron en "falsos" negativos/positivos, respectivamente. Describimos el rendimiento de cada aproximación, junto a sus medidas de "precisión diagnóstica" y las estadísticas de acuerdo.
Resultados En conjunto, las cinco aproximaciones identificaron 318-505 referencias para las 8 recomendaciones, de las cuales los revisores consideraron elegibles el 4,2 a 9,4% tras las dos rondas. Mientras que Pubmed superó a las otras aproximaciones (odds ratio de diagnóstico 12,5 versus 2,6 a 53), ninguna aproximación de búsqueda identificó por sí misma referencias elegibles para todas las recomendaciones. Individualmente, las búsquedas identificaron hasta el 40% de todas las referencias elegibles (n=71), y ninguna combinación de cualquiera de los tres enfoques pudo identificar más del 80% de ellas. Las estadísticas Kappa para la recuperación entre búsquedas fueron muy pobres (9 de cada 10 comparaciones pareadas no superaron el acuerdo esperado por azar).
Conclusiones Entre las plataformas de información evaluadas, Pubmed parece ser la más eficiente para actualizar este conjunto de recomendaciones. Sin embargo, la escasa concordancia en el rendimiento de las referencias exige que los grupos desarrolladores incorporen información de varias fuentes (probablemente más de tres) para este fin. Es necesario seguir investigando para replicar nuestros hallazgos y mejorar nuestra comprensión de cómo actualizar recomendaciones de forma eficiente.
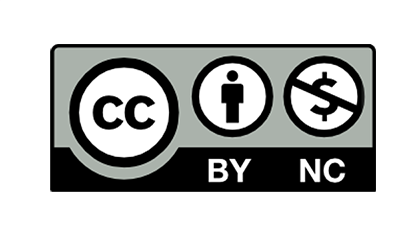