Comentarios
← vista completaPublicado el 2 de julio de 2019 | http://doi.org/10.5867/medwave.2019.06.7660
Ideas equivocadas frecuentes en estadística explicadas sencillamente
Common statistical misconceptions-plainly explained
This article aims to discuss the widespread and well-known problem of poor quality of statistical analyses in the biomedical scientific literature[1],[2],[3],[4],[5],[6]. Readers can make the best use of this article by taking the references and reading them in full. However, these excellent papers are not generally read by those who could gain benefit from so doing, perhaps because some are too long, too academic, or too mathematical. Whatever the reason, ultimately, these papers miss the public they intended to target. I am convinced that basic inferential statistics training should be imparted by non-mathematicians, who will be more sensitized to the common difficulties of students who are not gifted with numbers. In this article, I will explain some of these frequent errors or misconceptions in plain language, what is wrong, roughly why, and what could be done.
Census versus sampling
I will start elaborating on the simplest—although quite tricky—misconception I have encountered as a peer reviewer for medical journals. It is related to the misuse of statistical inference tools (i.e., hypothesis testing or confidence intervals) when analyzing data coming from a census procedure. According to the Merriam-Webster dictionary census is “a usually complete enumeration of a population.” The dictionary gives an example: “According to the latest US census, 16% of the population is of Hispanic or Latino origin.” Therefore, nobody should feel the necessity of calculating a confidence interval around 16%, since we know exactly the true value of the parameter of interest in the population.
The goal of inferential statistics is to discover some property or general pattern about a large group by studying a smaller group of people in the hopes that the results will generalize to the larger group. We rightly apply statistical inference because we take random samples, and we end up with estimates of the true parameters, which may be close or far from the true value of the population. We then apply statistical techniques that take into consideration this uncertainty, enabling us to generalize the results to the population of origin with a certain confidence.
Despite this simple reasoning, authors are reluctant to restrict their analyses to the descriptive statistics in accord with the design of the study. I daresay this reluctance comes—to some extent—from the difficulty researchers have when interpreting their results. Regrettably, many researchers rely on hypothesis testing to draw conclusions from their data. But, imagine a study that we run on the total population to assess the effectiveness of an intervention, and the observed effect size is, let’s say, 30%. That’s it. Next, all that is needed is to discuss the possible bias that may have crept into the design and conduct of the trial. Finally, you would discuss whether 30% is good enough or how it compares to alternative interventions or any other consideration regarding the impact of the results on the current knowledge of the topic of interest. Unfortunately, the fact that there are no p-values to help in the discussion and conclusion of the manuscript leaves many authors uneasy, facing the real question: what do the results mean?
Curiously enough, I have not found many references regarding this issue. I have asked professors of biostatistics from renowned universities from US and France, who confirmed what I have just explained, admitting that some statisticians feel perplexed when confronted with the situation of “no sampling, no uncertainty, thus no inference, no confidence intervals, no p-values.”
Separate p-values are not the way to compare groups
The second misconception I have chosen to explain here is the ubiquitous error that follows when observing a statistically significant change in X when A is present and not observing a statistically significant change in X when B is present; one may conclude mistakenly that the effects of A and B are different. This error has survived decades. Douglas Altman wrote about this in a 1991 book[7]. Among a list of “errors in analysis,” he points out the following: “performing within-group analyses and then comparing groups by comparing p-values or confidence intervals.”
In 2009, Watson et al. published an article of a clinical trial they conducted to assess the efficacy of a cosmetic “anti-aging” product[8]. In a letter to the editor, Martin Bland pointed out the many flaws he identified in the article, one of them being the one we discuss here. He went on to say: “For wrinkles at 6 months, the authors give the results of tests comparing the score with the baseline for each group separately, finding one group to give a significant difference and the other not. This is a classic statistical mistake. We should compare the two groups directly”[9].
Then there is the other common practice of the so-called “before-after” study design. This design consists of measuring a given variable of interest on a single group of subjects prospectively; first, at baseline and later at a specified point in time. With these data, you can compute for each subject the difference observed between the two time-points (baseline minus follow-up) thus obtaining the mean of all these differences that represents the mean change observed over time. A hypothesis test may then be performed comparing the mean of these differences against zero to estimate how likely it would be to observe such a difference when the null hypothesis is true. However, the “before-after” design does not include a control group, and many textbooks do not warn the reader on the many biases that this design entails. Graduate students sometimes start off using this simple, cheap, and easy design. Maybe the whole problem arises from teaching statistics in isolation when it should go hand in hand with methodology principles.
Reporting baseline statistical comparisons in randomized trials
Running hypotheses testing on every variable reported in the classical Table 1 of a manuscript that summarizes patient characteristics at baseline of a randomized clinical trial is unnecessary. Firstly, because this analysis is not addressing the research question and, secondly, because if randomization was used to allocate participants to the treatment groups, then the null hypothesis is true, by definition, for all baseline characteristics[5].
The Consolidated Standards of Reporting Trials (CONSORT) states this clearly in item 15: Baseline demographic and clinical characteristics of each group. The CONSORT reporting guideline is very clear when it addresses the issue of how to report baseline characteristics: “Unfortunately significance tests of baseline differences are still common … Tests of baseline differences are not necessarily wrong, just illogical. Such hypothesis testing is redundant and can mislead investigators and their readers. Rather, comparisons at baseline should be based on consideration of the prognostic strength of the variables measured and the size of any chance imbalances that have occurred”[10].
What can we do?
Why are statistical errors so prevalent in the biomedical published literature? One reason may be that there is a shortage of statisticians in peer review. Consequently, poor quality papers beset by statistical errors are continuously published[6] and the more they are out there, the more these misconceptions get picked up by readers believing they are scientifically and statistically sound. This state of affairs is unlikely to change in the short run. For peer review, journals should engage both experts who are knowledgeable in their clinical specialty, as well as in basic inferential statistics.
Notes
Competing interests
None declared.
Note from the editor
This commentary was originally submitted in English and Spanish.
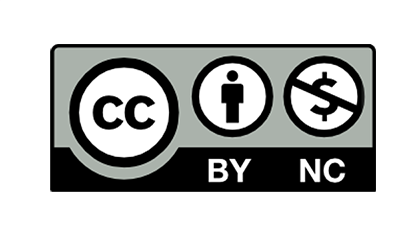