Methodological notes
← vista completaPublished on May 15, 2024 | http://doi.org/10.5867/medwave.2024.04.2790
Key factors in the choice of appropriate outcomes for clinical trials
Factores clave en la elección de desenlaces apropiados para estudios clínicos
Abstract
Health research is the foundation of medical knowledge and healthcare system recommendations. Therefore, choosing appropriate outcomes in studies of therapeutic interventions is a fundamental step in producing evidence and, subsequently, for decision-making. In this article, we propose three key factors for the choice of outcomes: the inclusion of patient-reported outcomes, since they focus on the patient's perception of their health status and quality of life; the consideration of clinically relevant outcomes, which are direct measurements of the patient's health status and, therefore, will be decisive in decision-making; and the use of core outcome sets, a tool that standardizes the measurement and interpretation of outcomes, facilitating the production and synthesis of appropriate evidence for the evidence ecosystem. The correct choice of outcomes will help health decision-makers and clinicians deliver appropriate patient-centered care and optimize the use of resources in healthcare and clinical research.
Main messages
- Choosing appropriate outcomes allows for the generation of useful knowledge for decision-making in healthcare.
- When choosing outcomes for research, it is key to consider clinically relevant outcomes, outcomes reported by patients, and those previously prioritized by a core outcome set.
- Clinical significance should be paramount when interpreting an outcome beyond statistical significance.
Introduction
Health sciences research is the foundation of medical knowledge and, to a large extent, healthcare system recommendations [1]. There is a long way from basic science to clinical practice for a candidate intervention (e.g., a molecule or a physical phenomenon) to become a clinically accepted and recommended treatment. The MAGIC (Making Grade the Irresistible Choice) foundation proposes an evidence ecosystem consisting of a cycle from evidence production, synthesis, dissemination to clinicians and patients, implementation, evaluation, and improvement, and then again to evidence production. Between each step, a handover of information lays the groundwork for the next stage. At the model’s core, five elements act simultaneously and in an integrated manner: reliable evidence, common understanding of methods, digitally structured data, tools and platforms, and the culture of sharing [2].
This transition from "knowing" to "doing" is the main objective of clinical research since evidence-based decision-making can be made only when this is accomplished. Given that the amount of research has been steadily increasing [3,4], different studies related to the same topic (disease or medical condition) must contribute to generating knowledge through consensus to improve the quality of care and health outcomes of patients. Uncoordinated interaction between researchers and decision-makers can lead to inappropriate research with suboptimal use of resources [5]. This is why a coordinated international work plan is required through scientific communities of interdisciplinary teams and patients or their representatives to prioritize research topics, avoid redundant work, and generate clinical recommendations with the highest possible level of evidence [6].
An important part of this coordinated action is choosing optimal outcomes to include in a clinical investigation. The GRADE (Grading of Recommendations Assessment, Development, and Evaluation) methodology classifies outcomes as "critical," "important," and "unimportant" according to the relative importance assigned to them by the recommendation panel. Thus, a critical outcome directly influences decision-making; an important one probably will, and an unimportant one will not [7].
This article is the thirteenth in a methodological series of narrative reviews on general biostatistics and clinical epidemiology topics, which explore and summarize published articles in user-friendly language in the main databases and specialized reference texts. The series is oriented to the training of undergraduate and graduate students. It is carried out by the Chair of Evidence-Based Medicine of the School of Medicine of the University of Valparaiso, Chile, in collaboration with the Research Department of the University Institute of the Italian Hospital of Buenos Aires, Argentina, and the Centro Evidencia UC, of the Pontificia Universidad Católica de Chile. This manuscript aims to discuss the key factors that should be considered by both authors and readers of scientific evidence when analyzing clinical research outcomes.
Why do we recommend a treatment?
In general, therapeutic interventions seek five objectives: to increase longevity, prevent comorbidities, reduce symptoms, improve quality of life, and optimize the use of resources [8]. Therefore, we will consider an intervention effective when it improves an outcome that reflects one or more of these objectives as directly as possible.
For example, the effectiveness of two therapeutic approaches for colon cancer in adults could be determined by measuring mortality, recurrences, occurrence of metastases, tumor size, tumor markers, microscopic bleeding, quality of life, pain, and adverse reactions, among others. Given that not all outcomes are likely to have the same impact on patients, families, professionals, and decision-makers, the fundamental question is which outcome(s) we should prioritize to measure in clinical research. Which outcome(s) are likely critical for healthcare decision-making? [9]. The importance of this question lies in the fact that the effectiveness of the studied interventions will be determined by measuring the selected outcomes. Therefore, a good outcome should be feasible to measure and considered relevant for decision-making throughout different contexts. In this article, we propose three key factors that every researcher should consider when choosing an appropriate outcome: the inclusion of patient-reported outcomes, the consideration of clinically relevant outcomes, and the use of core outcome sets.
First key factor: patient-reported outcomes
The adjudication of an outcome in clinical research refers to assessing the presence, absence, or change in the measurement of an outcome. Health outcomes can be assigned in different ways and by different adjudicators. For example, a change in plasma biomarker measurement through testing would be directly adjudicated by the laboratory, a clinician would adjudicate a change in tumor size through interpretation of imaging tests, and a change in pain intensity could be adjudicated by patients themselves or by an outside observer (caregivers or clinical staff) [10].
Patient-reported outcomes (PROs) are "reports of a patient’s health status that come directly from the patient, without any interpretation by a clinician or other person" [11]. Since they represent patients' perception of their health status, quality of life, and, ultimately, the most meaningful outcomes for them [12]. These outcomes respond directly to two of the goals for which we prescribe treatment: decreasing symptoms and improving quality of life. Thus, patient-reported outcomes generally provide crucial information for clinicians to make appropriate decisions [10].
Sometimes, there is a poor correlation between patient-reported outcomes and outcomes reported by laboratories, clinicians, or outside observers. For example:
-
There is a low correlation between forced expiratory volume and patient-reported quality of life in patients with chronic obstructive pulmonary disease [13].
-
In oncologic patients, the intensity of symptoms may be underestimated according to the interpretation of clinical staff [14].
-
In functional pathologies such as irritable bowel syndrome, pain syndromes, or symptoms such as nausea and fatigue, assessing a patient’s condition is limited and hardly objectifiable through external observation (reported by family members or other accompanying persons), clinical evaluation, or examinations [10].
As a general rule (but by no means mandatory), patient-reported outcomes should be prioritized in clinical research planning over those reported by third parties, with the notable exception of outcomes related to survival/mortality.
Second key factor: clinically relevant outcomes
When choosing a suitable outcome, it is essential to discern between a clinically relevant one and a surrogate one.
Mortality and quality of life will likely be high-priority outcomes for patients and clinicians involved in decision-making. An intervention that achieves decreased mortality with improved quality of life for colon cancer patients is likely to be considered a successful treatment. These outcomes are relevant since they represent a desirable state of health. On the other hand, the measurement of tumor size is not a relevant outcome in itself. Its importance lies in a causal inference or hypothesis. We measure tumor size because we indirectly assume that a smaller tumor size will lead to lower mortality and better quality of life. However, this causal reasoning is based on pathophysiological logic, which often does not behave linearly and directly in clinical practice.
A clear example of this occurs in the treatment of dyslipidemia. In patients with dyslipidemia, improving their lipid profile could be considered a relevant outcome. This is based on the theoretical assumption that a patient with dyslipidemia who improves his or her lipid profile will be less likely to die or to present a major cardiovascular event. In other words, we assume a direct and linear causal relationship between improved lipid profile with improved survival and decreased risk of cardiovascular events. However, this may not always be the case. For example, cerivastatin was withdrawn from the market for increasing mortality due to rhabdomyolysis despite the notable improvement in lipid profile [15].
The hypothetical example of colon cancer and the real example of cerivastatin in dyslipidemia lead us to the conclusion that there are two types of outcomes:
-
"Clinically relevant" outcomes are relevant for decision-making, as they directly measure a patient’s health status [16].
-
"Surrogate", " substitute", or "intermediate" outcomes are less relevant but are intended to predict or be associated with the former, representing an indirect measure of a clinically meaningful outcome that is used as a predictor in clinical studies [17].
Surrogate outcomes are related to the pathophysiological mechanism, being part of the biological process that causes the clinically relevant outcome to occur, so it is expected that they can be predicted through measuring biomarkers [16,17]. However, as we have already seen with cerivastatin, this is not always the case. Table 1 provides examples of studies on drugs such as rosiglitazone [18], torcetrapib [19], and fluoride [20], which showed a dissociation between findings measured by surrogate outcomes and clinically relevant outcomes.
From a mechanistic perspective, surrogate outcomes could be considered more important since they facilitate conducting clinical trials as they tend to be more accessible, cheaper, and faster [21]. However, to determine whether a treatment contributes to patients' well-being, we must know how it affects outcomes relevant to them [22].
We should note that a patient-reported outcome is not necessarily clinically relevant. For example, a patient might report mild pruritus that does not impact his or her quality of life in a chemotherapy treatment. In turn, the patient may not report a clinically relevant outcome, such as mortality or certain laboratory tests for specific pathologies (e.g., viral load in patients with human immunodeficiency virus, HIV).
Are surrogate outcomes bad?
While in theory, it is preferable to use clinically relevant outcomes because they provide better information to clinicians on when to use treatment [22], there are occasions when they may be difficult to access due to being more expensive, complex to measure, requiring prolonged follow-up or a larger sample size [16,23]. In cases where measuring a clinically relevant outcome involves excessive methodological and financial complexity, surrogate outcomes may be justified. For example, the measurement of glycosylated hemoglobin for patients with diabetes mellitus and viral load for HIV carriers are widely used surrogate outcomes because, due to the natural history of these pathologies, patients remain asymptomatic for an extended period before the appearance of potentially clinically relevant outcomes.
Third key factor: Core Outcome Sets
Due to the diversity of outcomes that can be heterogeneously prioritized among different research groups or decision-makers, reaching a consensus on a minimum set of outcomes relevant to measure in different contexts (which in GRADE terminology could resemble the concept of critical and important outcomes) is necessary. The Core Outcome Sets (COS) have attempted to generate these consensuses [24].
Core outcome sets are endorsed and standardized outcomes that should be measured and reported in all clinical trials for a given disease or health condition [25]. These have been compiled in specialized resources, such as the Core Outcome Measures in Effectiveness Trials (COMET) Initiative website [24]. For their development, a guideline of recommendations, the Core Outcome Sets Standards for Development (COS-STAD), has been outlined with three defined steps. The first step consists of defining the scope of the work, identifying the pathology, population, setting, and intervention to be investigated, and ensuring that there is no other work on the same topic in the COMET database. The second step is assembling an interdisciplinary panel composed of researchers, healthcare professionals, physicians, medical industry professionals, patients, family members, and/or caregivers. The third step involves identifying and prioritizing outcomes. Identification is done through primary or secondary qualitative studies that evaluate the outcomes reported in clinical trials. Prioritization is usually carried out using the Delphi technique, which consists of submitting a list of the relevant outcomes identified by the research team to the interdisciplinary panel so that they can give a score according to the importance they attach to them. Then, a second round is carried out, where the outcomes that have been unanimously considered irrelevant are eliminated. A new list is sent to the interdisciplinary panel with feedback and the score obtained in each outcome so that they can change their score if they consider it appropriate. This process is repeated until a consensus is reached, and ultimately, a final discussion of the results takes place [26,27].
For example, the COMET initiative website includes a study that investigated Core Outcome Sets for eczema [28], where the consensus outcomes to be prioritized in research on this topic were:
-
Clinical signs.
-
Symptoms.
-
Long-term control of eruptions.
-
Quality of life.
In this way, core outcome sets standardize outcome measurement, facilitating the production and subsequent synthesis of evidence appropriate to the evidence ecosystem [29].
Interpretation of outcomes: statistical significance vs. clinical significance
Once outcomes have been chosen or prioritized, proper interpretation of the results is essential for optimal clinical and public health decision-making. Therefore, it is important to understand the differences between statistical and clinical significance since a statistically significant outcome does not necessarily imply that it is clinically relevant, and vice versa [30,31].
Statistical significance refers to the probability that an outcome occurred by chance being very low. It is usually determined by convention as a "P value of less than 0.05" for a given statistical test or as a "95% confidence interval that does not cross the null value" [32,33]. In contrast, clinical significance is delimited by the minimally important difference, which marks the smallest change in patient-reported outcomes that patients perceive as important (either beneficial or detrimental) and might cause them and their treating physician to consider a change in therapy [34]. Clinical significance is determined by the accuracy of the results [35,36]. Suppose we set a minimally important clinical difference of 10 millimeters of mercury (mmHg) to treat arterial hypertension. Suppose a study’s confidence interval (CI), a measure of the results' precision, indicates that a treatment generates a blood pressure change of -20 mmHg, with a CI ranging from -25 to -15 mmHg. In that case, we can say that the treatment is estimated to lower blood pressure by a magnitude as high as 25 mmHg or as low as 15 mmHg. In both cases (even in the worst case), the treatment generates a clinically significant change in blood pressure (greater than the predefined minimally important clinical difference). This result is accurate since it does not cross the line of clinical significance. On the other hand, if the change were -10 mmHg with a CI ranging from -15 to -5 mmHg, we would have a more complex scenario. The difference would be clinically significant at best (-15 mmHg), but at worst (-5 mmHg), it would be clinically irrelevant. In this case, the CI crosses the clinical significance threshold and is, therefore, imprecise. In this manuscript, we will not delve into this specific issue, as it has been reviewed extensively in another article in this methodological series [36]. A correct interpretation of an outcome includes determining its statistical significance and clinical significance using an explicit minimally important clinical difference.
Applying to a research project
To better understand the key factors proposed for choosing an appropriate outcome, we will analyze the application of each to the hypothetical case of a group of investigators who must select the outcomes to be included in a study comparing two treatments for bronchial asthma.
First key factor: patient-reported outcomes
First, to have an overview, we can classify all possible outcomes to be measured according to the reporter, which are detailed in Table 2.
Also, some outcomes may be reported by more than one adjudicator. For example, the number of hospitalizations, emergency room visits, exacerbations, or asthma attacks can be reported by the patient, a family member, or the clinician.
As a general rule, it is advisable to include those outcomes reported by patients (such as those mentioned in Table 2) since they directly affect the perception of their health status and, therefore, will guide decision-making according to their results.
Second key factor: clinically relevant outcomes
Secondly, clinically relevant outcomes should be prioritized over surrogate outcomes unless the former are very difficult to obtain. Among the clinically relevant outcomes, we can find the quality of life, intensity, and frequency of symptoms or exercise tolerance since they are direct measurements of the patient’s state of health and will allow decision-making in their treatment.
Third key factor: Core Outcome Sets
We searched the COMET-initiative.org website to evaluate this factor and selected a study that developed a core outcome set for bronchial asthma evaluation [37]. The consensus outcomes included:
-
Asthmatic exacerbation.
-
Changes in asthma control (subjective perception, presence of symptomatology, and pre-bronchodilator forced expiratory volume value).
-
Quality of life as measured by standardized asthma surveys.
-
Number of asthma-related hospitalizations.
-
Emergency department consultations due to asthma.
To conclude, an appropriate set of outcomes for this question would be quality of life, symptom intensity and frequency, exercise tolerance, number of hospitalizations or emergency department visits, and number of asthma attacks or exacerbations since they are patient-reported or directly affect how the patient feels, are clinically relevant and are prioritized in core outcome set.
Final recommendations
An outcome that complies with the three aspects proposed in this article and that is interpreted considering clinically relevant differences will probably be relevant for decision-making in multiple scenarios. It will also allow for better production and utilization of evidence, which should be considered by researchers in order to optimize resources. Considering these key factors for prioritizing and interpreting outcomes contributes to the early stages of the evidence ecosystem (evidence production and synthesis). Consequently, it also contributes to better development of the next stages of the ecosystem (dissemination, implementation, evaluation, and improvement) so that healthcare decision-makers and clinicians can deliver appropriate quality of care while optimizing the use of healthcare and clinical research resources [38].
We propose the following recommendations for an appropriate choice of outcomes to evaluate when designing clinical research protocols:
-
Prioritize outcomes reported directly by patients (except for outcomes related to mortality/survival) to assess outcomes that directly affect patients' perception, quality of life, and/or well-being regarding their health condition.
-
Whenever possible, choose clinically relevant outcomes, whether reported by patients or not, since these favor adequate clinical decision-making.
-
Once the potential outcomes to be included in a study have been identified, review the Core Outcome Sets of the health condition on the COMET site or another related site to ensure that the essential outcomes defined by an interdisciplinary consensus for evaluating a health condition are included.
-
We suggest considering the inclusion of patients in the design of the protocol and the discussion of the results to generate an approach to the investigation of their own pathology when preparing primary experimental studies. We believe involving them in the early stages of clinical knowledge generation could be beneficial.
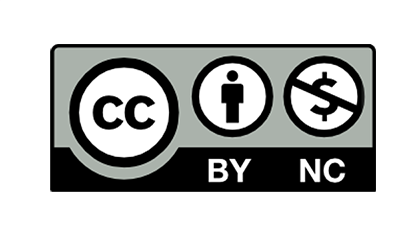