Notas metodológicas
← vista completaPublicado el 5 de junio de 2020 | http://doi.org/10.5867/medwave.2020.05.7930
Ensayos clínicos con análisis secuencial y su interrupción precoz ¿cómo interpretarlos?
How to interpret clinical trials with sequential analysis that were stopped early
Abstract
Sequential analysis of clinical trials allows researchers a continuous monitoring of emerging data and greater security to avoid subjecting the trial participants to a less effective therapy before the inferiority is evident, while controlling the overall error rate. Although it has been widely used since its development, sequential analysis is not problem-free. Among them main issues to be mentioned are the balance between safety and efficacy, overestimation of the effect size of interventions and conditional bias. In this review, we develop different aspects of this methodology and the impact of including early-stopped clinical trials in systematic reviews with meta-analysis.
Main messages
- Clinical trials could be finished early due to the efficacy of the intervention, its safety, or for scientific reasons.
- Sequential clinical trials stipulate repeated intermediate analyses in their methodological design in order to investigate intergroup differences in a set time frame. Sequential clinical trials allow continuous monitoring of emerging data to conclude rather sooner than later.
- Intermediate analyses raise the probability of type I error and could promote the adoption of therapy without fully understanding its consequences or overestimating the treatment effects.
- A meta-analysis of interrupted clinical trials may overestimate the treatment effects.
Introduction
In human research, the clinical trial is the primary experimental methodological design. It is a controlled experiment used to evaluate the safety and efficacy of interventions for any health issue and is used to determine other effects of these interventions, including analyzing adverse events. Clinical trials are considered the paradigm of epidemiological research for establishing causal relationships between interventions and their effects[1],[2],[3]. They are the primary studies that nurture systematic reviews of interventions that will provide an even higher level of evidence. Therefore, the characteristics of the clinical trial will directly affect the result of a systematic review and, eventually, its meta-analysis.
Clinical trials usually include a pre-planned time frame, concluding once the expected events for the participants occur. Both the time frame and the minimum number of participants that must be included in the comparison groups to show significant differences in clinically relevant outcomes are defined a priori. However, due to ethical conditions, the study may have to be terminated early, which may be for efficacy reasons (for example, undoubted efficacy is observed in one intervention over another), safety reasons (for example, multiple adverse events in an intervention) or scientific reasons (for example, the emergence of new information that invalidates conducting the trial)[4]. An example could be what occurred with the use of human recombinant activated protein C in critically ill patients with sepsis: The original clinical trial, published in 2001, was stopped early after an interim analysis due to apparent differences in mortality[5], which led to the recommendation of its use in the Surviving Sepsis Campaign guidelines[6]. Some subsequent trials raised concern because of the increased risk of severe bleeding[7], questioning the reduction in mortality found in the original study until the drug was finally withdrawn from the market in 2011 and removed from clinical practice guidelines[6]. As observed, early discontinuation is not without contingencies and controversies, although it can be included during the protocol of some clinical trials: sequential clinical trials[8].
In this narrative review, we develop the main theoretical aspects and controversies regarding the execution of clinical trials with a sequential design that leads to early positive discontinuation, as well as their impact on systematic reviews with meta-analysis in order to clarify some concepts that have been scantly treated in the literature. There are different sequential analysis: classic or fixed, flexible, and by groups[9]. In this review, we will cover the latter, the most widely used in clinical trials.
What are sequential clinical trials?
Sequential clinical trials stipulate repeated intermediate analyses in their methodological design in order to investigate intergroup differences over time, which has various advantages. Intermediate analysis can result in:
- The continuity of the study: shows no differences, continues with the inclusion of participants, and carries out another intermediate analysis.
- Interruption due to futility or equivalence: reaches a maximum sample size defined in advance and accepts the null hypothesis of equal effects in case the analysis does not show statistically significant differences.
- Interruption due to superiority or inferiority: demonstrates differences and concludes the trial through an interim analysis[8],[10].
Characteristics and analysis of their results
Sequential clinical trials must have a large sample size since intermediate analyses involve fractionating and comparing blocks of the sample. Likewise, they must span at least two years, and the intermediate analyses must be carried out by an independent data monitoring committee to take care of masking[4]. Thus, if a statistical rule indicates the need to stop the study, an interim analysis can be performed with the data collected to assess the emerging evidence on the efficacy of treatment up to the moment of stoppage[11]. Due to their dynamic nature, clinical trials that follow this methodology must evaluate outcomes that may occur in the stipulated period, as well as guarantee the rapid availability of data for their interim analysis. Sequential analysis is a method that allows continuous monitoring of emerging data to conclude as soon as possible while controlling for the overall error rate[12]. Developed by Wald in the late 1940s and adapted for the medical sciences years later, sequential analysis has been widely used since then and has opened a new chapter in large-scale studies by creating and using statistical and data monitoring committees[12],[13]. The existence of these committees is justified based on some disadvantages of this type of study, which will be addressed later. It is important to note that the decision to stop a clinical trial is more complex and is not only subject to an interim analysis adjusted by a statistical method.
Sequential designs provide a hypothesis testing framework for making decisions regarding the early termination of a study, sometimes making it possible to save expenditures beyond the point where the evidence is convincing about the superiority of one therapy versus another. One effect is that the number of patients who would receive an inferior therapy will be reduced. Another is that this new information to patients will be more quickly disseminated to health personnel, providers, and decision-makers[11].
Intermediate analyses: periodic evaluation of the safety-efficacy balance
Intermediate analyses that seek to prove a benefit of the intervention raise the probability of a "false positive" or type I error. This error occurs when the researcher rejects the null hypothesis even while it is true in the population. It would be equivalent to concluding that there is a statistical association between both arms when, in fact, the association does not exist. Consequently, the more comparisons between interventions are made, the higher the probability of making a type I error, for which some statistical adjustments should be considered[14]. Existing methods of making these adjustments include sequential stopping rules such as the O'Brien and Fleming limits or Haybittle and Peto (rules also known as group sequential analysis methods with completion rule), as well as the α expense generalizations of Lan-DeMets[15],[16]. In these last rules, it is possible to carry out the intermediate analyses that are desired without them having been previously established since there is a decrease in the value of statistical significance by avoiding making an associated error to multiple comparisons[4]. These methods are difficult to implement and require advanced statistical knowledge[17]. Although there are recent methodologies in evaluating sequential designs[18], classical methods have been widely accepted and implemented in practice[19]. However, multiple problems can arise when researchers stop a trial earlier than planned, mainly when the decision to stop the trial is based on the finding of an apparently beneficial treatment effect[20]. The problem of balancing safety and efficacy information is possibly the most difficult to solve. It is common for safety-related outcomes to occur later, and more infrequently than efficacy-related outcomes. A trial stopped early for its efficacy could promote the adoption of therapy without fully understanding its consequences, because the trial was too small or the duration was too short to accumulate sufficient safety criteria, a potential problem even if the trial continues until the planned term[12]. This happens because the sequential analysis is based on the efficacy of the interventions, so it has less power to evaluate their safety, as illustrated by the example on the aforementioned human recombinant activated protein C.
Special considerations for interim analysis
A) Conditional bias
Another problem associated with sequential analysis is that when the trial is stopped due to a small or large effect size, there is a greater chance that the trial is at a "high" or "low" level regarding the magnitude of the effect estimation, a problem known as "conditional bias"[12]. Bias may arise due to large random fluctuations in the estimated treatment effect, particularly early in the progress of a trial. Figure 1 shows the variability in the effect's estimation according to time in three possible theoretical scenarios. The upper red line shows a trial whose effect fluctuates towards an exaggerated effect. The lower red line shows a trial whose effect fluctuates towards exaggerated harm. The blue line shows a trial whose effect oscillates around the true effect. The interruption limits in each scenario could determine very different effects regarding the true effect depending on whether traditional threshold or interruption rules are used.
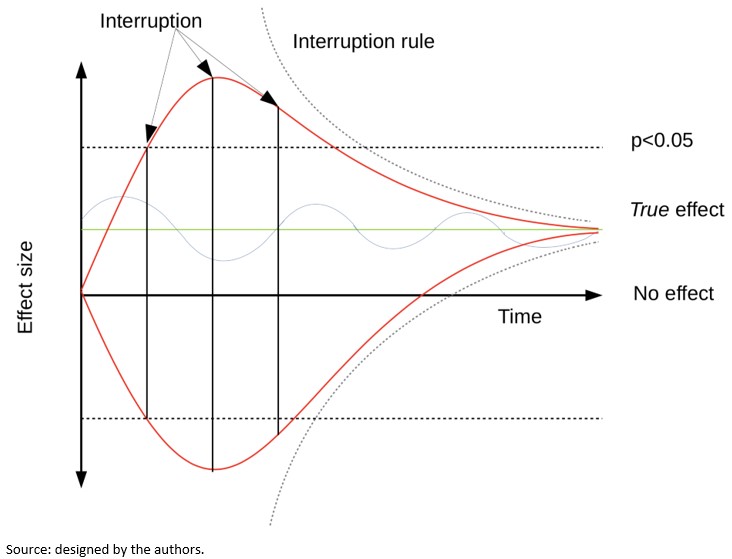
When researchers stop a trial based on an apparently beneficial treatment effect, its results may provide erroneous results[20]. It has been argued based on an empirical examination of studies stopped early that conditional bias is substantial and carries the potential to distort estimates of the risk-benefit balance and the meta-analytic effect. It has also been asserted that early discontinuation of a study is sometimes described with much hype, making it difficult to plan and carry out further studies on the same subject due to the unfounded belief that the effect is much more significant or safer than it is in actuality[12].
The probability of this conditional bias has been recognized for decades. Several methods of analysis have been proposed to control it after the completion of a sequential trial. However, such methods are less well known than methods for controlling type I error and are rarely used in practice when reporting the results of trials stopped early[19],[21].
B) Overestimation of treatment effect
Statistical models suggest that randomized clinical trials that are discontinued early because some benefit has been shown, overestimate the treatment effects systematically[20]. Similarly, by stopping early, the random error effect will be greater, since the number of observations will be fewer[8],[10]. By repeatedly analyzing the results of a trial, it will be the random fluctuations that exaggerate the effect that will lead to its termination: If a trial is interrupted after obtaining a low p-value, it is likely that, if the study was continued, the future p values would have been higher, which is associated with the regression to the mean phenomenon[14],[22].
Bassler and colleagues[20] compared the treatment effect of truncated randomized clinical trials with that of meta-analyses of randomized clinical trials that addressed the same research question but were not stopped early and explored factors associated with overestimation of the effect. They found substantial differences in treatment effect size between truncated and non-truncated randomized clinical trials (with a ratio of relative risks between truncated versus non-truncated of 0.71)—with truncated randomized clinical trials having less than 500 events regardless of the presence of a statistical interruption rule and the methodological quality of the studies, as assessed by concealment of allocation and blinding. Large overestimates were common when the total number of events was less than 200; smaller but significant overestimations occurred with 200 to 500 events, while trials with over 500 events showed small overestimates. This overestimation of the treatment effect arising from early discontinuation should be differentiated from any other type of overestimation due to selective reporting of research results; for example, when researchers report some of the multiple outcomes analyzed during research based on their nature and direction[23]. The tendency of truncated trials to overestimate treatment effects is particularly dangerous because it would allow for the introduction of publication bias. Their alleged convincing results are often published with no delay in featured journals and hastily disseminated in the media with decisions based on them, such as the incorporation into clinical practice guidelines, public policies, and quality assurance initiatives[24].
Implications for systematic reviews and meta-analyses
Given this rapid dissemination of truncated trials in mainstream journals[25],[26],[27], and their extensive incorporation into clinical practice, it is highly likely that, during searches of primary studies for systematic reviews, these studies are identified and included. Here, the aspects that should be adequately reported in the primary studies are the estimation of the sample size, the intermediate analysis that led to the interruption, and the rule that has been used to determine it. If systematic review authors do not notice truncation and do not consider early discontinuation of trials as a source of possible overestimation of treatment effects, meta-analyses may overestimate many of the treatment effects[28]. The inclusion of truncated randomized clinical trials may introduce artificial heterogeneity between studies, leading to an increase in the use of the random-effects meta-analytic technique, making it essential to examine the potential for bias in both study-fixed models and random-effects models[19].
In response to this risk, the exclusion of truncated studies in meta-analyses has been proposed. However, this exclusion generates an underestimation of the effect of the intervention that increases according to the number of interim analyses, which has been demonstrated through simulations[19]. This underestimation is fueled by the combination of two types of bias: estimation bias, related to how the effect measure is calculated, and information bias, related to the weighting mode of each study in the meta-analysis. When the proportion of sequential studies is low, the bias to exclude truncated studies is low. When at least half of the studies are subject to sequential analysis, the underestimation bias is in the order of five to 15%, regardless of whether the fixed or random effects approach was used. When all studies are subject to interim monitoring, the bias may be substantially higher than that range. Overall, these simulation results show that a strategy that excludes truncated studies from meta-analyses introduces a bias in the estimation of treatment effects[19].
On the other hand, although the studies stopped early would demonstrate an overestimated treatment benefit, it should not be too surprising that their inclusion in meta-analyses leads to a valid estimate. While it is true that, by including truncated trials in the meta-analysis, the difference observed in treatment overestimates the true effect, and that conditional to non-truncation, the difference observed in treatment underestimates the true effect, taken together, the effects of truncation and non-truncation would balance each other to allow an intermediate estimate. However, these simulations assume that the results of one trial do not influence how another trial is performed or whether that trial is directly ever performed[19]. This is unlikely to reflect reality since if a trial accidentally overestimates the effects of treatment and is therefore stopped early, it will be one of the first to be conducted and published and correction trials that would contribute to the combined estimation of a meta-analysis would never be carried out[24],[29].
Finally, the inclusion of trials concluded early could have implications for the certainty of the evidence. Since the number of events in these trials is usually fewer (precisely for stopping before reaching the required size), the confidence intervals of the estimator are usually wide, which leads to imprecision in the results of systematic reviews, making it highly likely that additional research has a significant impact on confidence in the effect estimates[30].
Conclusions
Sequential analysis of clinical trials can be a useful and interesting tool in terms of time and resources, but it is not without problems, such as conditional bias and overestimation of effect size. However, the combined effects obtained by meta-analyses that include truncated randomized clinical trials do not show a problem of bias, even if the truncated study prevents future experiments. Therefore, truncated randomized clinical trials should not be omitted in meta-analyses evaluating the effects of treatment. The superiority of one treatment over another demonstrated in an interim analysis of a randomized clinical trial designed with appropriate discontinuation rules, outlined in the study protocol and adequately executed, is probably a valid inference, even if the effect is slightly more significant than true, although the estimate derived from it may be imprecise.
Notes
Authors contribution
Funding
Conflicts of interests
Ethics
From the editors
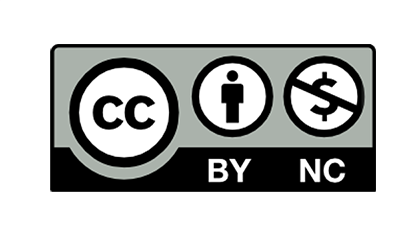